The UC Berkeley research team recently released its latest research result - the TULIP (Towards Unified Language-Image Pretraining) model. The model aims to improve the performance of visual language pre-training, especially in vision-centric tasks requiring high-fidelity understanding, overcoming the limitations of existing contrast learning models such as CLIP.
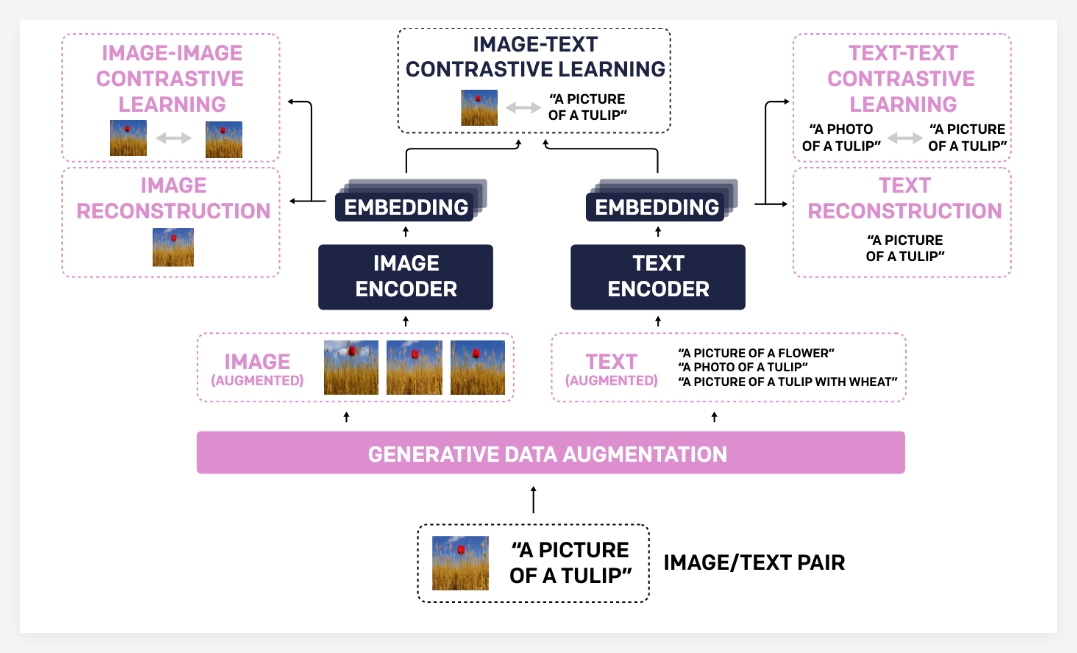
TULIP significantly improves the alignment between vision and language through innovative technologies such as integrated generative data augmentation, enhanced contrast learning, and reconstruction regularization . Experimental results show that TULIP has achieved state-of-the-art performance in multiple benchmarks, setting a new benchmark for zero-sample classification and visual language reasoning.
The reason why the TULIP model has achieved such significant progress is mainly due to its unique technology portfolio:
- Generative Data Augmentation : TULIP uses generative models to augment training data, thereby improving the robustness and generalization capabilities of the model. By synthesizing more diverse image-text pairs, the model can learn more comprehensive visual and linguistic knowledge.
- Enhanced Contrastive Learning : Unlike traditional contrast learning methods, TULIP not only focuses on the matching between images and text, but also introduces image-image and text-text comparison learning goals . This enhanced contrast learning method can help models better understand visual similarities between different images and semantic associations between different text descriptions, thereby improving their understanding of fine-grained information.
- Reconstruction Regularization : In order to further strengthen the alignment of visual and language features, TULIP adopts a reconstruction regularization strategy. This method enables the model to reconstruct the corresponding text description from the image features or the corresponding images from the text features, thus forcing the model to learn deeper cross-modal associations.
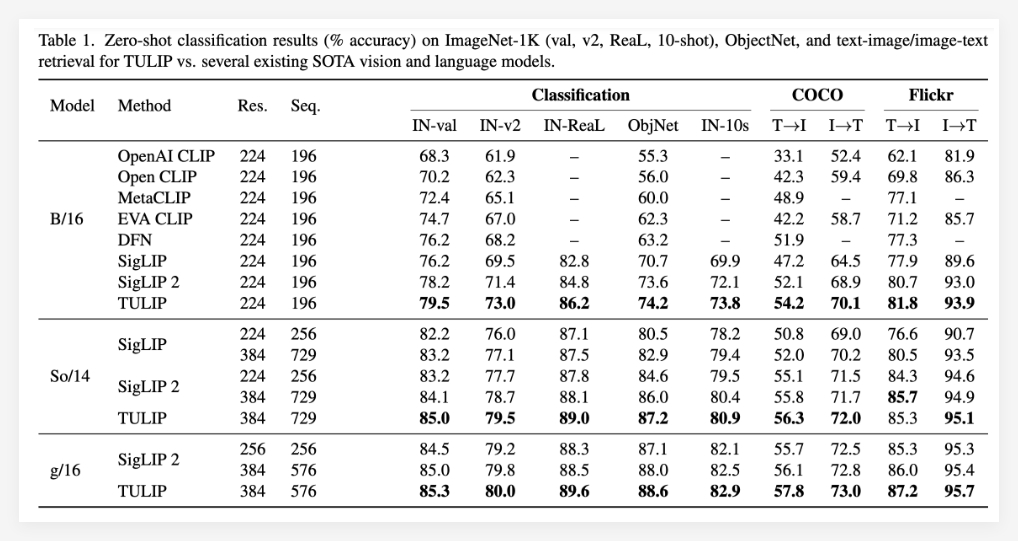
The experimental results fully prove the superiority of the TULIP model. TULIP has reportedly achieved current optimal levels in multiple important visual and visual language benchmarks (state-of-the-art) . Specific manifestations include:
- ImageNet-1K zero-sample classification significantly improves : TULIP can still accurately classify images without undergoing any specific category training, showing strong zero-sample learning ability.
- Enhanced fine-grained object recognition capability : TULIP can more accurately distinguish objects with nuances in images, which is crucial for application scenarios that require precise identification.
- Improvement of multimodal inference score : TULIP shows higher accuracy and stronger understanding in tasks that require inference in combination with image and text information.
It is particularly worth mentioning that compared with existing methods, TULIP has achieved up to 3 times performance improvements in MMVP benchmarking, and has also achieved 2 times performance improvements in fine-tuned visual tasks. These data fully demonstrate the great potential of TULIP in improving model performance.
Project: https://tulip-berkeley.github.io/